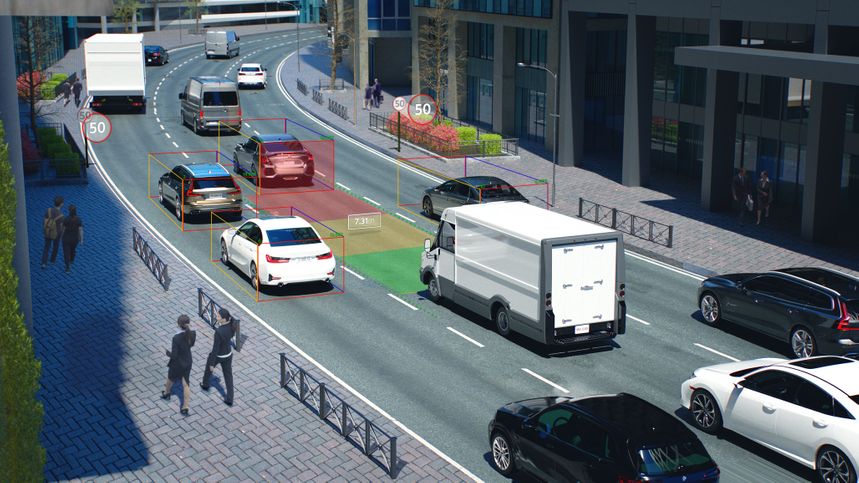
The idea for collision avoidance systems (CAS) has been around since the 1950’s and the concept is straightforward – warn drivers of an impending collision, allowing them to take preventative action or even to let the system actively assist the driver, for instance automatically braking to avoid a crash.
Actual implementation of these systems, however, is quite complex and it was only in the mid-90’s that they could be brought to mass market as an option for new vehicles.
Later, this technology was adapted as an aftermarket accessory. Since then, many fleets have discovered and adopted these retrofit collision avoidance systems for a number of reasons. For older vehicles, these retrofit devices are a way of equipping fleets with advanced technology without needing to purchase new vehicles. For new vehicles, retrofit collisions avoidance can sometimes be more cost effective than OEM equipment.
Of course, manufacturers have taken great pride in touting the strides made in OEM collision avoidance but what about retrofit devices? What new technological developments are now available for fleets seeking an aftermarket solution? There actually have been great strides made in improving these retrofit collision avoidance systems but since these developments are not really noticeable at first glance, let’s take a peek under the hood.
There are a number of different approaches to how collision avoidance systems sense objects, these include radar systems, lidar systems and cameras. Each system has advantages and disadvantages, including the ability to function in adverse conditions and cost.
But no matter what the sensing technology used, the key element is the artificial intelligence (AI) underlying a collision avoidance system. After all, what good is sensing an object unless it can be identified and the time to collision calculated?
Over the past several years AI has undergone a revolution with the development of deep neural networks (DNN) to replace the more traditional machine learning of the past. What’s the difference between the two?
The best-known explanation uses the example of a system programmed to create one database of photos containing dogs and another database of photos containing cats. In the traditional machine learning method people would have to go through a large sample of photos manually defining the rules of what is a dog and what is a cat, building a ruleset for the computer to determine whether the image it detects is a dog or a cat. With a DNN model you simply feed the computer images and tell it whether the image is that of a dog or a cat. The DNN then creates its own rules to classify these images. The end result is that the DNN is far more accurate than machine learning.
However, there are barriers to using DNN. One of the big challenges is that you need massive amounts of data in order for the system to construct a ruleset. Going back to our dog and cat example, you would have to enter an enormous number of examples into the network in order for it to develop rules.
For collision avoidance, the upside of DNN is enormous. A system leveraging DNN would be able to identify a wider range of potential hazards, identify them more accurately and from a further distance.
The recently released Mobileye® 8 Connect™ is the company’s first retrofit system designed to take advantage of DNN technology. The collision avoidance system is more accurate and can “see” vehicles at almost twice the distance of its predecessor. It can also identify vehicles, cyclists and pedestrians at night*.
One important advantage Mobileye has in bringing DNN to the world of collision avoidance is the company’s close relationship with many leading vehicle manufacturers. Over 25 of these companies use Mobileye technology in their new vehicles, giving Mobileye access to over 200 million(!) miles of high-resolution video from pre-production testing, the kind of massive dataset needed by DNN.
Another technology that has grown significantly over the years is telematics – telecommunications technology for vehicles. It is this technology that allows fleet management systems to keep track of vehicles’ locations, hours of service (HOS) and even maintenance needs.
And now collision avoidance systems are joining the telematics revolution – giving fleet managers access to driver safety information. Generally, this information focuses on data picked up by the G-Sensor (sometimes called an accelerometer). This sensor detects sudden vehicle movements, thus indicating to fleet managers when a vehicle has braked, accelerated or cornered sharply – incidents that may indicate poor driving habits.
Mobileye 8 Connect takes the telematics revolution one step further by not only tracking incidents recorded by the G-sensor but also those recorded by the system’s safety alerts:
- Headway Monitoring & Warning (which may indicate tailgating)
- Forward Collision Warning
- Pedestrian and Cyclist Collision Warning
- Lane Departure Warning
Recording these alerts (along with when and where they occurred) facilitates the production, for fleet managers, of detailed, comprehensive reports on driving behavior, how it can be improved and when it should be rewarded. And with the ability to incorporate system upgrades via the cloud (over-the-air updates), the analytical tools available to managers will be improved over time.
There is little doubt that with recent advances in AI and telematics today’s fleet managers have a powerful toolkit enabling them to make their fleets smarter, safer and more efficient than ever.
For more information on fleet safety and the new Mobileye 8 Connect, contact Mobileye.